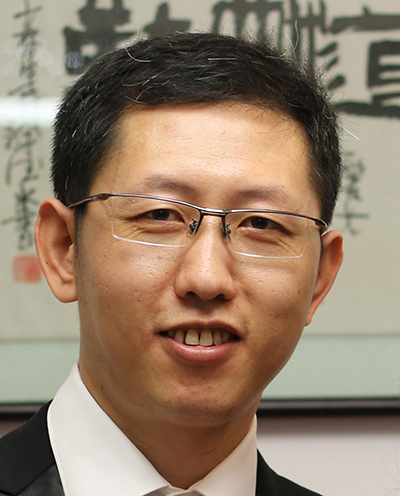
Chunming Lu’s lab in Beijing Normal University has published a paper titled “Shared neural representations of syntax during online dyadic communication” in NeuroImage. This is another fNIRS hyperscanning study within a month (check out another paper by Dr Xianchun Li and his group: https://www.alivelearn.net/?p=2172)! Lu’s lab studied the brain response of real time verbal communication between two people. We interviewed Dr Lu and his team and they are generous in sharing their experience about this study.
Congratulations on your recent paper in NeuroImage.
First of all, I would like to thank Dr. Xu Cui for giving me a chance to introduce our recent work at such a good place. Our work has benefited a lot from Dr. Cui’s pioneering work on fNIRS hyperscanning. His work has inspired a lot of studies in the past few years.
1. Reading your past publications I get a feel that you are very interested in studying the mechanism of human verbal communication. Why are you interested in this area?
My previous research mainly focused on the relationship between brain and language. I have been studying communication disorders such as stuttering for many years using fMRI. Stuttering is a common speech production disorder that affects about 1% of the general population. One of the major features of stuttering is that the typical symptoms of stuttering occurs more often in a dialog context. In a monolog or covert speech context, however, most people who stutter don’t stutter at all. Thus, it is suggested that social interaction play a key role in the relationship between brain and language. Since then I am keen to studying language (including verbal and nonverbal) communication in a social interaction context.
2. Since the experiment requires the participant to read, did the mouth or head motion introduce any artifact (noise) in the NIRS signal? If so, what did you do to remove/minimize it?
Speaking (and gesturing) will definitely introduce artifacts in the fNIRS signal. It is generally agreed that artifacts can only be avoided but can’t be removed. Fortunately, from our experiences, most of the artifacts resulted from movements can be avoided if you place the probes on the head appropriately.
But if artifacts do occur, what should we do? In our experiment, we have conducted a running-window procedure to identify the suspected artifacts. The percentage of time points that were contaminated by artifacts was less than 5%. We also conducted a simulation experiment by adding a variety of percent of artifacts to the signal (i.e., 5%, 10%, 15%, and 20%). The results showed that 15% artifacts would significantly decrease the detectability of the syntactic-related effect in our study. We also replaced the identified artifacts by means of the neighbor time points. The results of artifact-free data were the same as the raw data. These results indicate that data may not be significantly contaminated by artifacts when the artifacts are less than 5%.
There are several other good methods that can be used to deal with the artifacts issue. For instance, Dr. Cui has developed a nice method by calculating the correlation between the HBO and HBR signal. My colleague Dr. Haijing Niu also developed a software to do quality control, http://www.nitrc.org/projects/fcnirs.
3. This study is fairly big – you had 90 pairs of participants! What method did you use to recruit so many participants? Did you pay them? How long did it take to collect the data?
Thanks to BNU so that we could recruit so many participants ! Because psychology and cognitive neuroscience is one of the best disciplines of BNU, most students have interests and are willing to participant in psychological experiment. We also have some participants from other universities that are close to BNU.
Our participants were paid after the experiment. For each pair of the participants, the set up and performance of the experiment took about 1 hour in total.
4. You used wavelet transform coherence method to analyze the NIRS data. In your opinion, what is the advantage of wavelet over other methods?
I am aware that several different methods have been used to characterize interpersonal neural synchrony during social interaction. But so far WTC is one of the most popular methods among them, since its first application in Cui et al., 2012, NeuroImage.
WTC is my favorite because it provides both temporal and frequency information based on a relatively simple principle. During language communication, people usually produce 5-6 syllables per seconds, and they take turns in a dialog within a time window of less than 80-100 ms. Thus, a precise estimation on the temporal pattern is necessary for smooth communication. WTC allows us to take a close look at the temporally dynamic process of coordination during communication across many different frequency bands.
On the other hand, I also believe that there is no such a method that can address any issues. I would love to try different methods in different contexts.
5. How did you identify which frequency range/band to use to calculate the coherence?
What advice do you give to researchers who want to use fNIRS hyperscanning but have little experience?
Frequency selection has been considered as one of the key processes in fNIRS hyperscanning study. For studies that employed a traditional ER or BLOCK design, usually there is a priori hypothesis about the frequency of interest (FOI). For studies on naturalistic communication, however, so far there is no standard procedure for frequency selection.
During the past few years we have developed a procedure to address this issue (Zheng et al., 2018, HBM; Liu et al., 2019, NeuroImage). The idea is that the FOI should be defined based on a center and a range. The center should be a statistically strict threshold that determines the position of the frequency, whereas the range could be a relatively loose threshold that determines the width of the FOI. In Liu et al., 2019, the center was set as P < 0.0005 whereas the range was P < 0.05. All frequency ranges that survived this criteria were examined. In addition, the frequency ranges that totally overlapped among conditions were combined, whereas those differing in frequency position or range were considered independently.
Compared to EEG or fMRI, fNIRS is more friendly and easy to use. I believe that all people who have a good academic training are able to use it in their research.
6. Can you use one sentence to summarize your finding in this study?
A distinctive pattern of interpersonal neural synchronization underlies shared representations of syntax between interlocutors.
7. What is your plan for future research?
In the future, my research will continue to focus on the neural mechanism of language communication. We also have interests in how language is used in different social contexts, and how language is related to other aspects of cognition during social interactions.